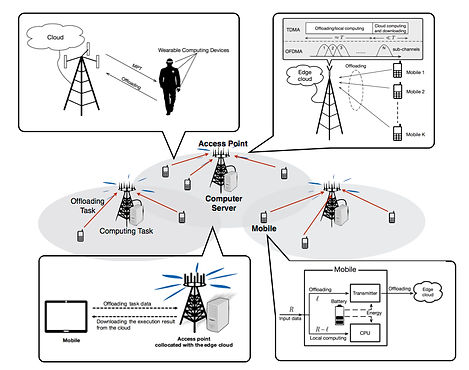
In next-generation networks, ultra-dense edge devices (ranging from base stations to TV set-top boxes) will be deployed at the network edge. Leveraging the abundance of computing resources at these edge devices, called mobile edge computing (MEC). The main feature of MEC is to push mobile computing, network control and storage to the network edges so as to enable computation-intensive and latency-critical applications at the resource-limited mobile devices such as sensors and IoT devices. MEC promises a dramatic reduction in latency and mobile energy consumption, tackling the key challenges for materializing the 5G vision. The promised gains of MEC have motivated extensive efforts in both academia and industry on developing the technology. The main thrust of MEC research is to seamlessly merge the two disciplines of wireless communications and mobile computing, resulting in a wide range of new designs ranging from techniques for computation offloading to new network architectures. A comprehensive survey of this research area can be found in [MEC1]. Our group contributes to this area by developing advanced computation-offloading technologies such as wirelessly-powered MEC, joint radio-and-computational resource allocation, live prefetching, and mobile cooperative computing. Moreover, we also conducted pioneering research on modeling and analyzing large-scale MEC networks, leveraging useful tools from stochastic geometry and queuing theory. Our selected work is summarized as follows.
-
Wireless Powered Mobile Computing: A novel framework of wirelessly powered mobile cloud computing has been proposed in [MEC2] for enabling computation in passive low-complexity devices such as sensors and wearable computing devices. A set of policies are derived to optimize the computing performance of two mobile operation modes, namely local computing and computation offloading, under the energy harvesting and deadline constraints.
-
Energy-Efficient Resource Allocation and Live Prefetching: In [MEC3], we investigate the resource allocation for a multiuser MECO system based on TDMA/OFDMA, accounting for both the cases of infinite and finite cloud computation capacities. A so-called MEC offloading priority function is derived to show a simple threshold based structure of the optimal resource allocation policy. The work is extended in [MEC4] to design energy-efficient resource management for asynchronous MECO, where different mobiles have heterogenous data-arrival time instants and deadlines. A novel architecture of live prefetching for mobile computation offloading is proposed in [MEC5] that enables prefetching based on task-level computation prediction and its simultaneous operation with cloud computing. The fetching policies are designed to minimize mobile energy consumption under a deadline constraint, enabling real-time control of the prefetched-data sizes of candidates for future tasks. Last, an opportunistic computation offloading is investigated in [MEC6] and [MEC7] to harvest idling computation resources at dynamic helpers by exploiting the non-causal and causal helper's CPU state, respectively.
-
Large-Scale MEC Networks: In [MEC8], we made the first attempt to construct a stochastic-geometry model for a large-scale MEC network and study its network-constrained latency performance, featuring spatial random distribution of network nodes, wireless transmissions, parallel computing at servers. Based on the model and under network performance constraints, the latency for communication and computation are analyzed by applying theories of stochastic geometry, queueing, and parallel computing. The results yield useful guidelines for MEC network provisioning and planning.
-
Over-the-air Computation: To support future IoT networks featuring dense sensor connectivity, a technique called over-the-air-computation (AirComp) was recently developed which enables a data-fusion to receive a desired function of sensing-data from concurrent transmissions by exploiting the superposition property of a multi-access channel. We aim at further developing the AirComp technology for next-generation multi-antenna multi-modal sensor networks to enable parallel multi-function computation via spatial multiplexing and accurate reception of the results by exploiting spatial diversity. In particular, a comprehensive framework for MIMO AirComp that consists of beamforming optimization and a matching limited-feedback design is proposed in [MEC9]. The framework was extended in subsequent work to wirelessly-powered AirComp system [MEC10], where the beamformer was jointly optimized with the wireless power control to further reduce the AirComp distortion, and massive MIMO AirComp system [MEC11], where a reduced-dimension two-tier beamformer design was developed by exploiting the clustered channel structure to reduce the channel-feedback overhead and signal processing complexity.
[MEC1] Y. Mao, C. You, J. Zhang, K. Huang and K. B. Letaief, "A Survey on Mobile Edge Computing: The Communication Perspective," IEEE Commun. Surveys Tuts. vol. 19, issue. 4, pp. 2322-2358, 2017. (ArXiv)
[MEC2] C. You, K. Huang and H. Chae, “Energy Efficient Mobile Cloud Computing Powered by Wireless Energy Transfer”, IEEE J. Sel. Area on Commmu., vol. 34, no. 5, pp. 1757-1771, May 2016. (ArXiv)
[MEC3] C. You, K. Huang, H. Chae and B. Kim, “Energy-Efficient Resource Allocation for Mobile-Edge Computation Offloading”, IEEE Trans. on Wireless Commun., vol. 16, no. 3, pp. 1397-1411, Mar 2017. (ArXiv)
[MEC4] C. You, Y. Zeng, R. Zhang, K. Huang, "Asynchronous Mobile-Edge Computation Offloading: Energy-Efficient Resource Management", IEEE Trans. on Wireless Commun., vol. 17, no. 11, pp. 7590-7605, Sep. 2018. (ArXiv)
[MEC5] S.-W. Ko, K. Huang, S.-L. Kim and H. Chae, "Live Prefetching for Mobile Computation Offloading", IEEE Trans. on Wireless Commun., vol. 16, no. 5, pp. 3057-3071, May 2017. (ArXiv)
[MEC6] C. You and K. Huang, “Exploiting Non-Causal CPU-State Information for Energy-Efficient Mobile Cooperative Computing”, accepted to IEEE Trans. on Wireless Commun.. (ArXiv)
[MEC7] Y. Tao, C. You, P. Zhang, K. Huang, "Stochastic Control of Computation Offloading to a Helper with a Dynamically Loaded CPU", submitted to IEEE for possible publication. (ArXiv)
[MEC8] S.-W. Ko, K. Han and K. Huang, "Wireless Networks for Mobile Edge Computing: Spatial Modeling and Latency Analysis", IEEE Trans. on Wireless Commun., vol.17, no. 8, pp. 5225-5240, June 2018. (ArXiv)
[MEC9] G. Zhu and K. Huang, "MIMO Over-the-Air Computation for High-Mobility Multi-Modal Sensing,” accepted to IEEE IoT Journal.. (ArXiv)
[MEC10] X. Li, G. Zhu, Y. Gong, and K. Huang, "Wirelessly Powered Data Aggregation for IoT via Over-the-Air Functional Computation: Beamforming and Power Control", submitted to IEEE for possible publication. (ArXiv)
[MEC11] D. Wen, G. Zhu, and K. Huang, "Reduced-Dimension Design of MIMO Over-the-Air Computing for Data Aggregation in Clustered IoT Networks,” submitted to IEEE for possible publication. (ArXiv)